Zegami Looks To Make the Big Problem of Big Data a Thing of the Past
The company provides a way to look into content stored within an existing archive system
Story Highlights
In an age of data overload — often big-data overload — the ever-increasing challenge is to take that mass of data, understand it, and apply it to create valuable content. And, more critically, how can you take an existing archive or managed assets and get a better understanding of what you have and what it means to your organization, without having to start from scratch, re-ingesting and recataloging? That is where Zegami comes in.
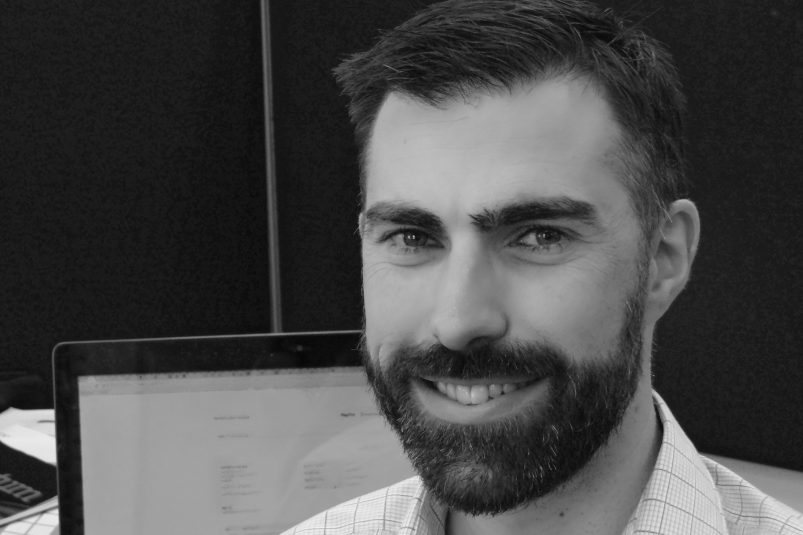
Zegami’s Roger Noble says the company is focused on unlocking content via easy-to-use search.
“As a sports-program producer or editor, you need to view all your available images or video and make timely decisions by tapping into those archives so that you can extract truly relevant material,” says Zegami CTO Roger Noble. “The needs of an archive changes over time, and machine learning is going to start to unlock those archives. But you need to be able to do searches in a smarter way that also gives emotional context to your content, something that was previously not possible.”
Zegami’s strength, he adds, is combining artificial intelligence more closely with relevant real-time data by using visualization to explore it in a much more practical way. And sports and broadcast media, he notes, need to make decisions as quickly as possible.
“Our premise is to unlock content and make it easy for someone without a technical background to embrace artificial intelligence and be able to explore that content quickly,” says Noble.
Many believe that artificial intelligence holds the key to next-generation data demands. The challenge for all involved is that machine learning, for all its buzz, is still difficult to use and requires someone to be quite technical in order to use it.
Zegami’s platform provides a single-screen interface that can tap into a variety of metadata and content fields, according to Global Marketing Director Oliver Abadeer. And, although company growth was spurred by the application of its platform to big-data analytics — finance, healthcare, human resources, schools — the broadcast industry has become a major focus: broadcast technology can be easily integrated into existing archive, storage, and asset-management systems.
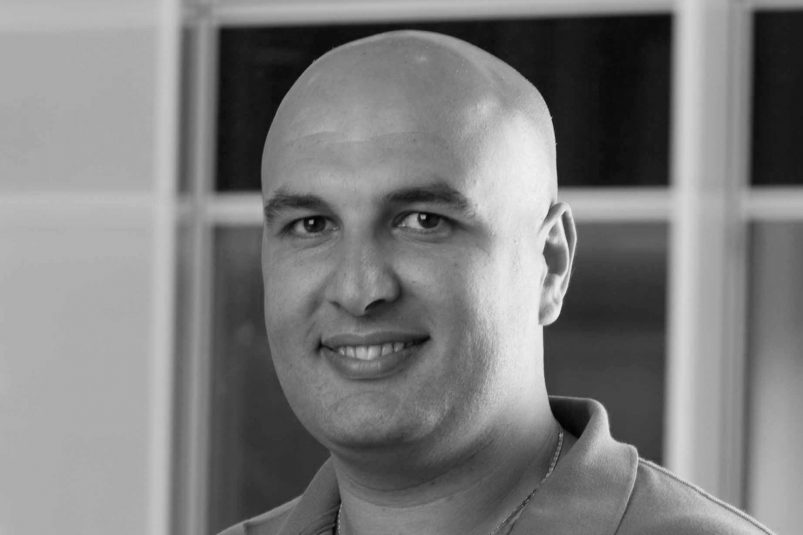
Zegami’s Oliver Abadeer sats the sports industry is facing a tsunami of data and needs easier systems to make use of it.
Abadeer says that Zegami’s offering of a tool for both content search and analysis in the same platform is unique. The goal is to save customers’ starting from scratch with their archive or asset-management system by layering Zegami’s platform on top. Via APIs and webhooks, it taps into the existing archive and live systems to provide a new level of insight and the ability to find relevant content “in the moment.”
This visual way of presenting data is a paradigm shift in the way people can look for content. The platform uses visuals — graphs, scatter plots, media tiles — that can be lassoed to find comparable data — for example, correlations, outliers, patterns, and relationships — incredibly quickly. Users can quickly figure out what their audiences want to see there and then, without having to work their way through lists or search engines or have a researcher do any “ground work” before the program airs.
“Zegami is a simple tool that is disruptive in terms of its search capabilities but not disruptive to any existing workflow,” says Abadeer. “There are some big broadcasters who simply don’t want to invest in yet another UI and MAM system and start over, and that is where we will be strongest.”
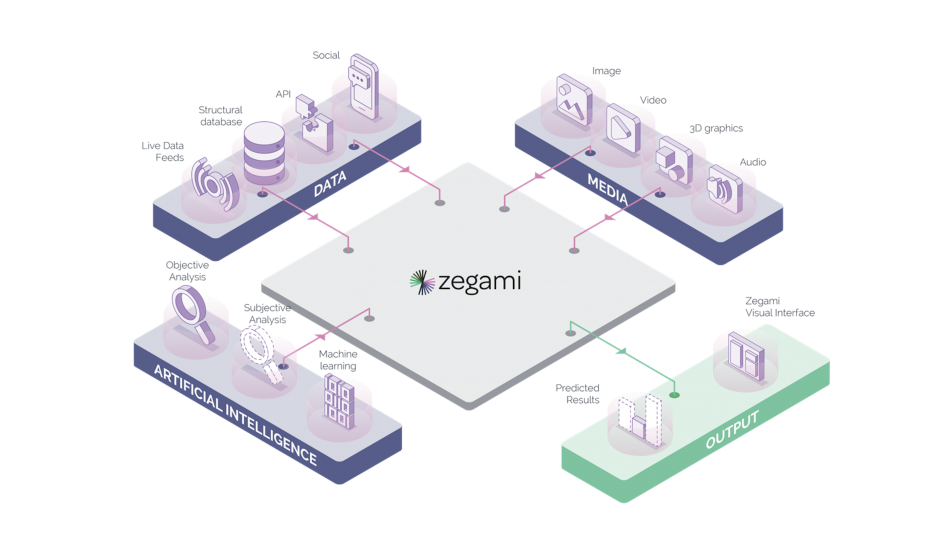
Zegami’s platform sits at the center of disparate systems and content.
One Zegami success story involves a Major League Baseball club that was struggling with how to gather all the notes and material from various scouts. The scouts would pull together information on upwards of 15,000 high school and college players and evaluate whether they were worth drafting. With Zegami, the scouts were able to combine their notes so that the management team could more easily compare players and make the best moves in the MLB draft.
“If a player they were looking at was drafted, they could quickly drill down and find other players that were similar but might not have been on their list,” Abadeer explains. “In the live environment of news or sports, any production team wants to react to something trending around an event and find the content most relevant to that point in time.”
Noble explains that, when Zegami integrates with an existing media-asset–management system, all the content remains within the MAM. The goal is not to set up a completely new system but rather to create a new way to look into the content stored within the system and make it easier to find.
Adds Abadeer, “In sports, there is a tsunami of unstructured data and a need to monetize assets in real time. People are looking not just to find content but also to analyze it in terms of engagement, and our tool allows us to do that.”
The role of machine learning to analyze sports-related content is critical to speeding up the discovery process. But there are limitations: machines are still learning to read emotions within a play or event. Zegami’s system can make it easier to connect subjective data to objective data like scoring, timing, etc. If AI and human sentiment are combined, the data can be fully optimized to give emotional context for live audience consumption.
The need for new ways to discover content, whether in deep archives or recent archives, is crucial, Noble says. New social-media platforms and services from Amazon and Netflix (as well as countless apps) reshape the ability to monetize content.
“We want to empower and enable users to be able to make decisions that can tap into these new technologies,” he points out. “There is a massive amount of unstructured content, and it is a problem that isn’t going to go away. So we’re here to fix it.”